Modeling
-
Standard Modeling
-
We used a gradient boosting model, incorporating various factors like location, square footage, and more to predict property prices
-
-
Image Modeling
-
Currently, the state of the art (SOA) models for Computer Vision task utilize Convolutional Neural Networks (CNNs).
-
Current SOA approaches utilize pretrained models such as VGG16, ResNet, Inception, or EfficientNet
-
-
To combine the models:
-
Transfer Learning:
-
Remove the classification layers from the original pretrained models, keeping the feature extraction layers and weights
-
Pass house images through the feature extraction layers of the pre-trained CNN to obtain a feature vector
-
-
Incorporate the feature vector along with the other independent variables (location, size, number of bedrooms, etc.) into a regression model
-
Model Evaluation
-
Evaluation of our model performance using our primary criteria for model performance, median percentage error. Our gradient boosting model using all features performed the best.

Sample Images used in Model
-
Our Model took advantage of both "real" and "rendered" images
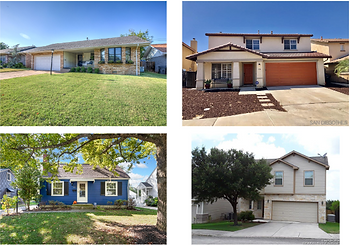
Incorporating Image Data into Model
-
Initial trial with Gradient Boost Model
-
Extract Features with EfficientNetV2L
-
Join features to dataframe
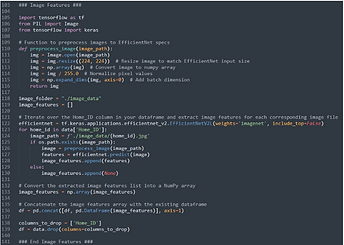